Artificial intelligence is not just a trend; it’s the key ingredient for success in businesses. Incorporating AI into process management unlocks new opportunities to optimize operations, reduce costs, and make fast, accurate decisions.
Among its many tools, machine learning stands out as a technology that enables systems to learn from data and drive continuous improvement. Discover how to implement it in your organization!
What is Machine Learning?
Machine learning is a branch of artificial intelligence that allows systems to learn from data to improve their performance without being explicitly programmed.
Its use in process automation reduces management time and costs, enhances decision-making, and transforms organizational productivity.
Use Cases of Machine Learning in Businesses
Discover some popular applications of ML in business management:
Loan Approval
One of the most common ways to use machine learning in business processes is for decision recommendations.
In this case, a pre-trained algorithm analyzes the entered data and makes a recommendation based on past decisions. Since the final decision is made by a human and not the algorithm, this is a low-risk way to incorporate ML into your processes, especially for critical decisions like bank loan approvals.
In such cases, it is helpful for the algorithm to be explanatory, so the person receiving the recommendation can understand the rationale behind the decision. To ensure success with this strategy, it is important to make users aware that the responsibility for decisions lies with them and not the algorithm.
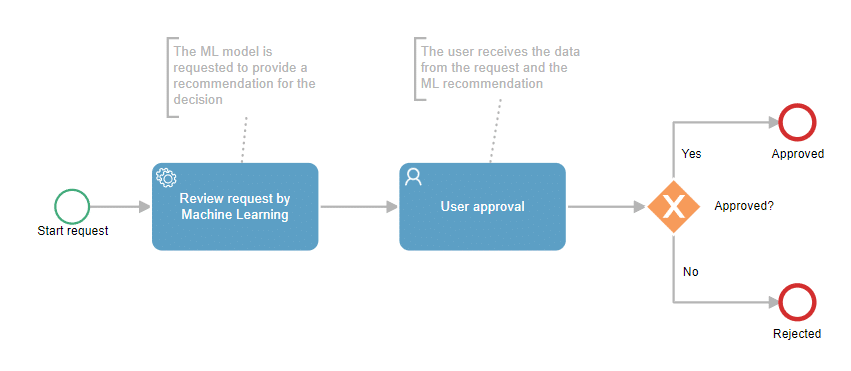
Candidate Screening in Recruitment
In recruitment and hiring processes, receiving a large number of resumes often makes it difficult to thoroughly analyze each one. This can result in highly qualified candidates being overlooked simply because their resumes weren’t adequately reviewed.
Incorporating machine learning into recruitment processes can provide benefits such as accelerating candidate screening and facilitating the identification of top talent.
One way to achieve more efficient resume screening is through the use of OCR techniques to extract text from resumes. Additionally, natural language processing techniques can be used to summarize the extracted text. This streamlines the review process by highlighting the most relevant qualities of each candidate.
Candidate Classification
Another strategy to incorporate machine learning in HR processes is decision-making.
There are several ways to apply AI-based decision-making to processes. For instance, a recruitment team can benefit by integrating an additional evaluation step to decide whether to accept or reject a candidate.
This approach addresses the potential consequences of a false positive—hiring an unqualified candidate—which could result in financial losses and customer dissatisfaction. To mitigate this, a manual evaluation can be performed only if the algorithm recommends accepting the candidate, as shown in the following example:
Purchase Requests
Machine learning algorithms can provide information about their confidence level in a decision, for example, indicating a 60% probability that a purchase request should be approved. This aspect is critical, as the confidence level of a decision varies significantly between 60% and 95% probability.
This information can be used to automate decision-making only if the algorithm’s confidence level exceeds a predetermined threshold, such as 90%. Otherwise, the decision is manually evaluated. This can be seen in the process illustrated in the following image:
Voting-Based Decisions
BPM tools, such as Qflow, enable decision-making through voting. Decisions can be made by multiple machine learning models and members of the organization. This approach reduces the risk of errors in the decision-making process.
The following image illustrates an example of a voting-based process:
Customer Retention
Maintaining good relationships with customers is essential for any organization. Losing a customer can have a significant impact, both financially and reputationally. To prevent this, many companies use machine learning models to assess the risk of customer churn.
For example, a mobile phone company looking to identify customers at risk of leaving can use a machine learning model for predictions. The model analyzes historical customer data, such as usage patterns, call durations, billing information, complaints, and late payments, to identify patterns. If the model indicates a high risk of churn, preventive measures can be taken to retain the customer.
In this process, the machine learning model evaluates updated customer information and generates risk predictions. If the model identifies a customer with high churn risk, an investigation phase is initiated. The company’s sales team may proactively contact the customer, offering special discounts, personalized plans, or other incentives to encourage their retention. Finally, the results of the measures taken are evaluated.
Fraud Detection in Banking Transactions
Another example that combines machine learning with BPM is detecting fraudulent transactions in banking. In this case, machine learning models can be trained to identify suspicious patterns and behaviors in banking transactions.
It is essential to choose an appropriate algorithm for this scenario, as effectiveness is not the only criterion. The algorithm must also be explanatory, enabling the bank to provide solid arguments if a suspicious transaction is blocked.
Additionally, suspicious transactions can be sent for manual review. This allows a detailed investigation of the transaction, ensuring an informed decision about its legitimacy. Manual reviews are conducted only when fraud is suspected, ensuring a smooth user experience by avoiding unnecessary delays or inconvenience.
Contact Request Optimization
On a product’s commercial website, the contact request form is crucial for interacting with interested visitors. However, handling the large volume of messages received daily can be challenging for the team.
By using machine learning in organizational processes, a model can be developed to analyze messages sent through the contact form. The model examines message content, detects patterns, and evaluates the relevance of each request. This enables the automatic filtering of irrelevant messages, allowing the team to focus on meaningful inquiries.
Machine learning can also classify contact requests by type, streamlining the process even further. For example, if a message refers to a technical inquiry, it can be automatically directed to the support team. Similarly, demo requests can be sent to the sales team responsible for coordinating product demonstrations. This enhances customer service processes and improves response quality, providing a satisfying experience for both the organization and its clients.
Decision Sampling
One way to evaluate a model’s effectiveness without requiring user actions is to randomly define whether a decision should be reviewed. A bonus of this approach is that decisions validated manually can be used to train and improve future model predictions.
How Do We Use Machine Learning in Qflow?
BPM tools like Qflow allow for the progressive and controlled integration of machine learning models into processes. This is possible because processes can be designed so that humans can oversee the decisions made by machine learning models. Once the results are sufficiently reliable, the process can be adjusted with simple changes to remove the need for human intervention. If necessary, processes can be reverted immediately without requiring programming efforts.
The following image illustrates how, by simply modifying the decision points for “Use machine learning?” and “Require human supervision?”, the desired behavior can be achieved.
Additionally, business processes provide valuable information for training machine learning models. For instance, if someone approves a purchase request, they may view a form containing amounts, reasons, suppliers, and other details. All this information, along with the decision made, can be used to train the machine learning model, enabling it to learn patterns and generate decision predictions. Thus, Qflow continuously collects information to improve ML algorithms, learning from the dynamic changes in business environments.
Benefits of Using ML in Businesses
Integrating machine learning into process management not only enhances organizational efficiency but also improves interactions with customers and anticipates future challenges.
Here are the main benefits:
- Process Automation: ML is a crucial tool for automating processes, reducing workloads for teams, and enabling them to focus on strategic activities.
- Improved Decision-Making: Through continuous learning, ML models generate accurate predictions and recommendations to support more informed decisions.
- Anomaly Detection: ML can be trained to identify risks, fraud, or problems in real time, allowing for timely resolutions.
- Reduced Operational Costs: By shortening task resolution times and identifying errors and failures, businesses can significantly cut costs.
- Adaptability and Scalability: Machine learning implementation enables systems to evolve alongside business needs, adapting to new challenges.
Challenges to Overcome
While it is intuitive to think that AI can help optimize business processes, many organizations face difficulties in adopting it.
Some common challenges include:
- Insufficient data to create effective AI solutions.
- Lack of trust in the decisions or predictions made by AI.
- Changes in employee workflows.
However, having automated processes with a BPM tool enables the natural and controlled adoption of AI.
Conclusion
Implementing machine learning transforms data into strategic decisions, automates processes, and enhances the experiences of both customers and employees.
Ensure your adaptability in the ever-changing and competitive business landscape by embracing innovation to stay ahead of the market. Try Qflow today and empower your processes with machine learning!